Solutions
Based on state-of-the-art and proprietary artificial intelligence and machine learning methodologies and algorithms, we deliver three types of solutions.
Clinical Development
optimization
Trial enrichment for faster recruitment and higher generalizability
Relaxing RCT inclusion/exclusion criteria based on Real-World Data to speed-up recruitment and improve result’s generalizability while maintaining probability of success.
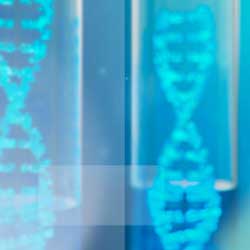
Modeling Huntington Disease progression to de-risk Phase III trials
Modeling Huntington’s disease progression heterogeneity allows to de-risk Phase III trials while optimizing their probability of success by targeting rapidly progressing patients.
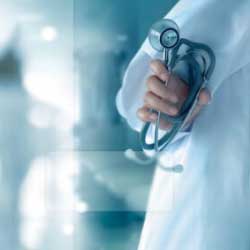
Adaptive trial design with historical control to optimize prevention of mother-to-child HIV transmission
A Bayesiann adaptive trial with a historical control was designed to accelerate and de-risk trial and to optimize prevention of mother-to-child HIV transmission.
Market Access /
HEOR & Pricing
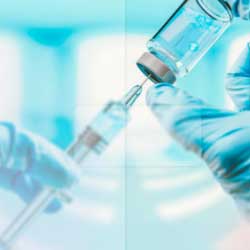
Predicting effectiveness from efficacy for an asthma drug to ascertain medical value towards regulators and payers
Building a predictive real-world disease model of asthma by integrating trial efficacy and drivers of efectiveness to predict the effectiveness of a new anti-asthma drug.
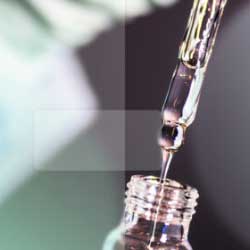
Modeling care and disease progression to optimize switching time in heart failure
Predicting the long term effectiveness of various treatment sequences to optimize switching strategies and therefore maximize medical value and care efficiency.
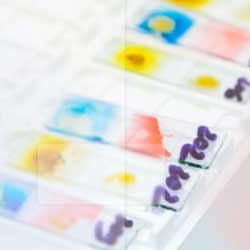
Predicting long-term outcomes from short-term endpoints to demonstrate drug value in rare disease (sIBM)
The link between symptoms and long-term outcomes is critical for regulators and payers, but difficult to substantiate and even more so for rare, slowly progressing and chronic illnesses like Sporadic Inclusion Body Myositis (sIBM).
Asset Lifecycle
Value Management
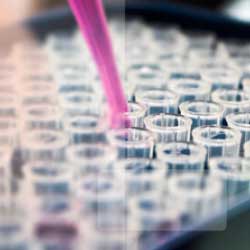
Identifying high-value PsA patients along disease progression to support portfolio value-based positioning
Characterizing high-value (high costs / high response rates) patients across disease stages by modeling disease progression onto structural damage under care pathways scenarii to maximize medical benefit and portfolio value.
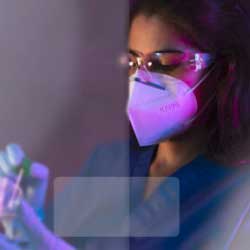
Pricing agreement based on long-term real-world cardiovascular outcomes in France
Modeling and predicting the real-life impact of a lipid-lowering drug on cardiovascular morbidity and mortality allowed to maintain reimbursement in France (risk sharing). Results had a positive impact on sales volumes and prices in multiple other countries.
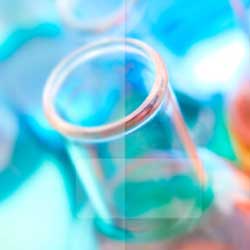
Forecast sales and patients flows under various positioning scenarii in a highly dynamic market
The context was the launch of a new drug in metastatic castrate-resistant prostate cancer, a highly competitive indication then. Real-world data-driven care pathway modeling and simulations provided value-based arguments for market access planning and payers negotiations